Modeling the fluctuation of word meanings using a mathematical model
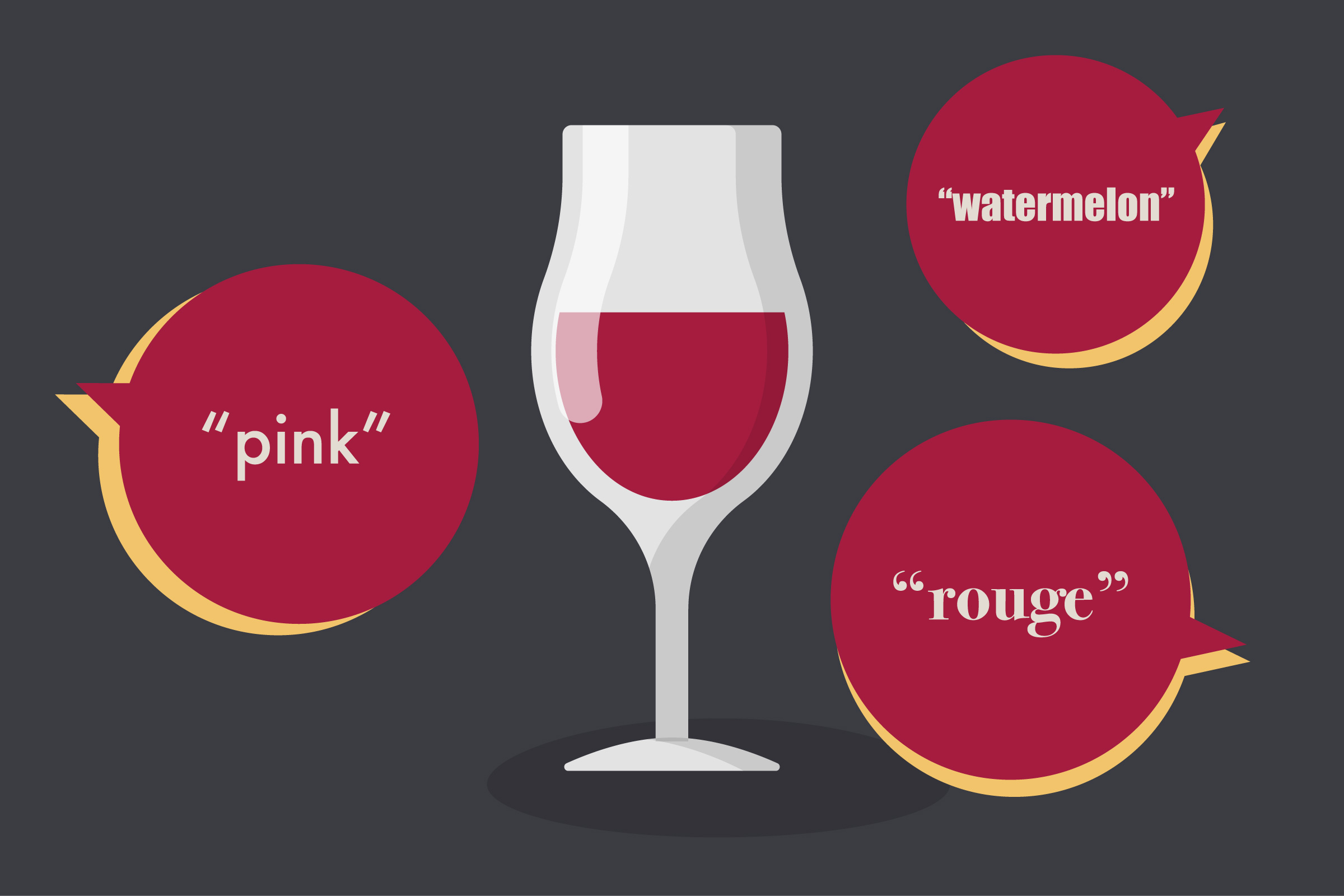
UTokyo-IIS researchers will introduce their research.
Fluctuate
We communicate using language, but the meanings of words fluctuate depending on who use them, when, where, and to whom they are used. Associate Professor Naoki Yoshinaga has been engaged in research on “adaptive” natural language processing (NLP) for computers to accurately understand and manipulate the living language we use.
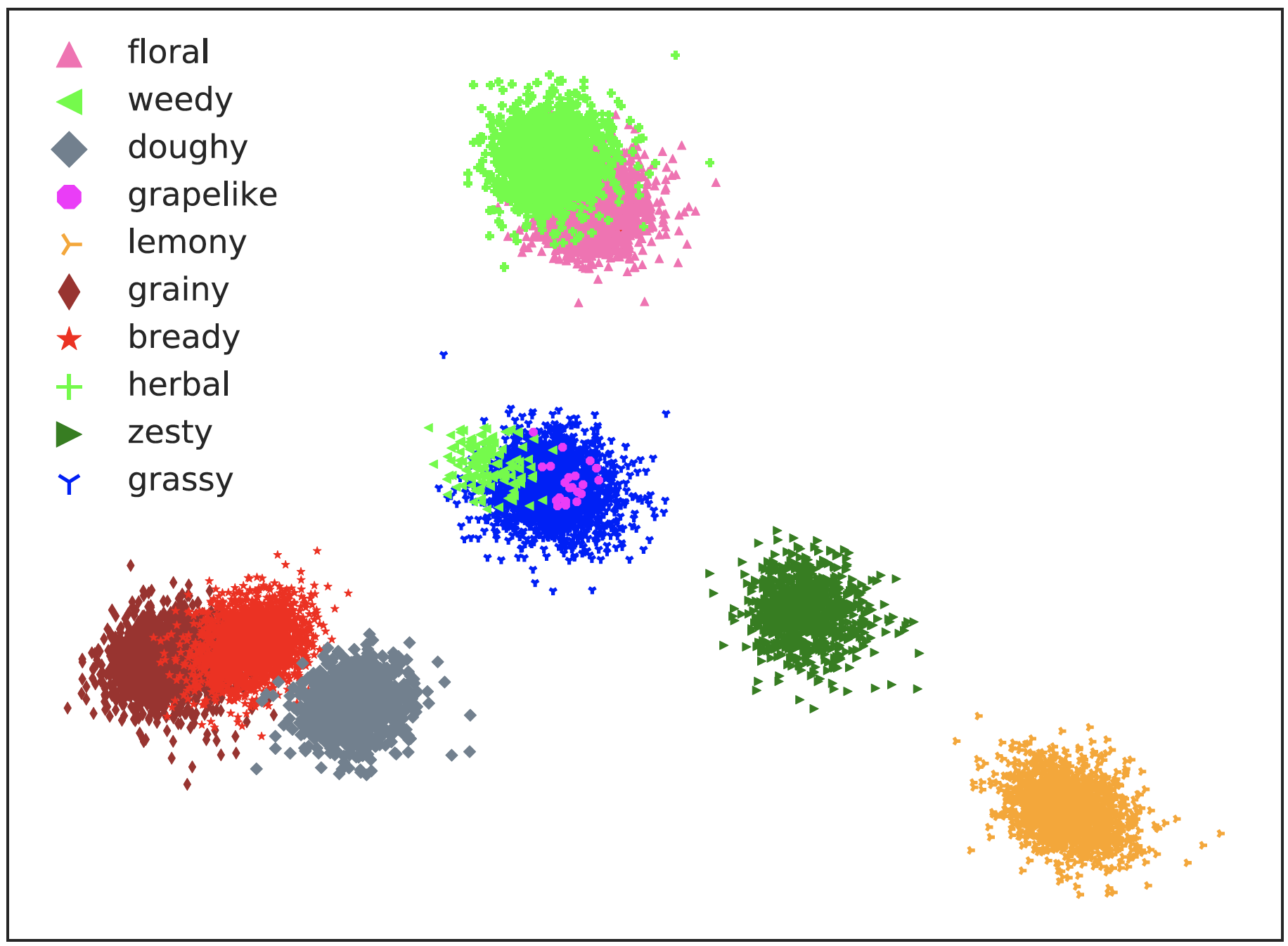
A visualization of words used by individuals writing craft beer reviews (Oba et al. Personal Semantic Variations in Word Meanings: Induction, Application, and Analysis. Journal of Natural Language Processing, 27(2). 2000)
This scatter plots visualize the meanings of words used to express taste in craft beer reviews, learned as vector representations for each individual who used the words, in a two-dimensional space as points (this study has been done with students (the first author: Daisuke Oba, who has obtained PhD in 2023)). All the words show individual variations in meaning; for instance, the meanings of “herbal” (+) and “weedy” (◀︎) differ in the degree of meaning fluctuations, and some individuals use the same word, such as “weedy,” with a significantly different meaning with the others, as seen in the point in the upper right. Furthermore, different words, such as “bready” (★) and “grainy” (◆), are used to express the similar meanings by individuals, highlighting the ambiguity inherent in the meanings of the words we use.
Currently, large language models (LLMs), which are actively studied in NLP and enable conversational AI like ChatGPT, are trained to capture the average behavior of language from aggregated vast amounts of text written by various people. Roughly speaking, LLMs can be seen as having learned to capture the average meaning of words (the center of meanings represented by words of the same color in the plot above). As a result, while LLMs can yield satisfactory outcomes for most users, they cannot be the optimal technology for each user. In fact, obtaining desired responses from LLMs often requires detailed instructions.
Our lab’s goal is to personalize the language technology to individual users, similar to kana-kanji conversion and predictive text input. Specifically, we are conducting research on mathematical modeling of languages that mimics individual’s language competence and evolves autonomously. Conversely, if we can realize models that mimic individual’s language competence, analyzing these models as in the above scatter plots helps us understand how we recognize and comprehend words (and ultimately, the world). This could allow us to recognize discrepancies in language perception with native speakers while learning a second language, or to understand our latent biases regarding various topics. Meanwhile, by using models that mimic each other during conversations, we may be able to “translate” our words into the words of the others, helping to resolve discrepancies in the meanings we convey.
Oba et al. Personal Semantic Variations in Word Meanings: Induction, Application, and Analysis. Journal of Natural Language Processing, 27(2). 2000
DOI: 10.5715/jnlp.27.467
Text:Associate Professor Naoki Yoshinaga
Comments
No comments yet.
Join by voting
How did you feel about the "Possible Future" depicted in this article? Vote on your expectations!
Please visit the laboratory website if you would like to learn more about this article.
Share